Building Biotech with Brains: Strategies for Maximizing Value of AI-Driven Biotechnology Inventions
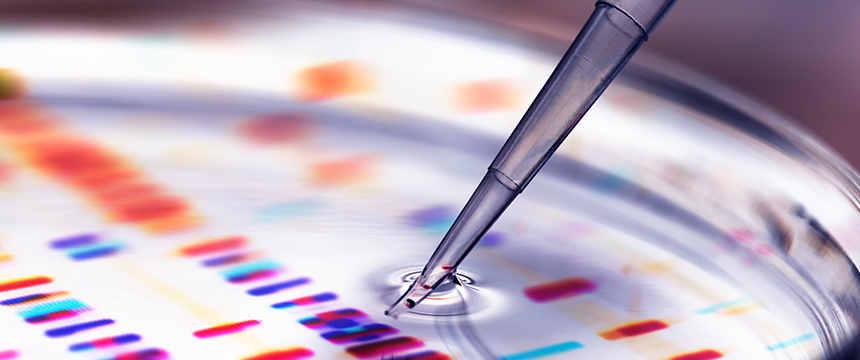
The rapid rise of artificial intelligence (AI) and machine learning (ML) in biotechnology products and services is becoming a driver of the personalized medicine and health care sectors. While this integration can require special consideration during development of a patent portfolio, stakeholders across engineering, legal, and executive teams in both established companies and start-ups can leverage it to create valuable intellectual property (IP) assets in the marketplace. Technology and legal teams in particular can work together to develop stronger IP that applies machine learning applications to biotechnology, with an R&D process and marketplace analysis that is aware of its unique IP pressure points.
Identifying the Nexus Between Biotechnology & AI/ML
When building a robust patent portfolio and broadly capturing potential IP assets, stakeholders should approach the convergence of high-tech/biotechnology holistically. That is, IP managers should consider all potential implementations of the integrated ML/biotech asset in order to build out and cover the technical objectives underlying the commercial embodiments. Although inventors may focus on any one particular embodiment, IP portfolio managers should analyze assets from a wider lens. While this may be true across the technical spectrum, the holistic viewpoint becomes particularly important on the frontier of new technologies involving both high-tech and biotechnology components.
Product development processes can capture important IP insights early with targeted inquiries about the technology itself. Consider whether there are multiple potential data inputs that could yield a useful output metric. For instance, a particular metric for a disease could be evaluated based on the presence of particular genetic markers or by the accumulation of misfolded proteins. Generally, all data sets capable of yielding an objective metric when integrated into the machine learning model could be the basis for valuable IP.
Processes and models to analyze potentially unique data can also yield strong IP. If an asset relies on a process for obtaining unique data that enables you to train a model faster and with more accuracy, focusing various patents on generation and management of the data can protect from a competitor that gains access to similar data in the future.
Navigating prohibitions on patenting abstract ideas under the Alice framework can be critical when the platform is based on improvements to the ML/AI models themselves. Courts have consistently interpreted Alice to favor claims focused on improvements to the tangible concepts and disfavored claims directed solely to algorithms. Companies should focus on how the technology gathers or integrates the raw data, as well as novel physical elements that result in technical improvements to any process or product. This should extend beyond the preferred commercial embodiments that will be covered by your claims.
Building a Patent Portfolio for AI-Driven Biotech that Maximizes Competitive Advantage
Whether a business is primarily focused on the AI components or the biotechnology components can inform its strategic direction for creating impactful patent assets. For example, many pharmaceutical companies center their IP assets on the products and methods yielded as a result of using an AI/ML model, while in contrast many biotechnology startups base their business platform on particular ML models and data driving those models rather than on any one particular output. Further, companies interested in drug discovery or novel therapeutics may utilize their ML or AI asset as an analytical tool for converting raw data into a useful metric for disease or a new drug candidate. In this case, there may be multiple machine learning models capable of producing a therapeutically or diagnostically useful metric based on the raw data.
Each of these strategic directions can require distinct technical and legal analyses:
- Where the output data provides a useful metric – for example, the likelihood of successful treatment or novel drug candidates – companies may want to devote resources to protecting the specific output delivered using the AI solution as opposed to the AI solution itself.
- When the business focus is on the particular output generated, providing as many models and examples as possible in a patent application can maximize value.
- Where the AI solution can be applied to a broad range of actions and insights, the IP focus should be on the model and data input. Examples might include advanced ML systems capable of analyzing and finding patterns in genes or biomarkers indicating the presence of a disease and/or likelihood of successful treatment.
Ultimately, the strategic direction and R&D processes for developing high-value and impact IP for AI-driven biotechnology can be informed by the positioning of the company in the market and specific features of company products and services at the nexus between AI and biotechnology.
AI in Health Care Series
For additional thinking on how artificial intelligence will change the world of health care, click here to read the other articles in our series.